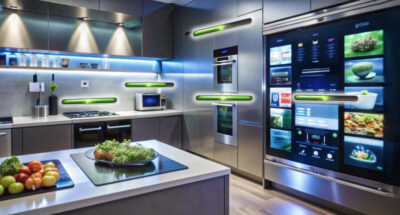
How a new generation of negative electricity prices creates opportunities for consumers
Subsidies for renewables have led to electricity prices frequently falling to less than zero, creating opportunities for consumers. ...
by Ralf W. Seifert, Richard Markoff, Matthew Spooner Published 5 August 2024 in Strategy • 6 min read
Devising a KPI to capture the accuracy of a demand plan is a knotty problem, even though it might seem intuitively simple. After all, there is a forecasted value for the demand for a given period (usually monthly) and the actual sales, which are well-known after the fact. Compare them, and there you go.
However, building out a KPI definition quickly leads to several complications. First, you need to decide which demand plan to use. Using the most recent will usually lead to the highest accuracy since predictions are easier for the short term than for the long term. But for supply chains with high latency marked by long lead times, the most recent demand plan will not accurately reflect the risks to the business posed by poor demand plans.
There are also aggregation issues, covering how to capture the accuracy of a group of Stock Keeping Units (SKUs) – unique identifiers used to track individual products rather than just one SKU. The approach taken to resolve the aggregation question represents a bifurcation point that lumps demand planning KPIs into two broad categories.
In the first category, each SKU is measured independently and then aggregated by some sort of average. The averaging method can come in different flavors, such as weighted/not weighted, or the units may be in volume or sales revenue. This category of demand planning KPI is referred to as demand planning accuracy.
The second group of KPIs falls under the rubric of forecast bias. Over the past 20 years, several organizations have considered forecast bias as an important measure. In simple terms, forecast bias is the tendency to systematically over or under-forecast, leading to higher inventory or worse service levels. There is therefore an inherent tendency to over-forecast – you are unlikely to be fired for too much inventory, but to deliver poor service on a major product launch will leave you searching for a new job. 
Sales teams, particularly in tough economic times, also tend to introduce positive forecast bias: it’s far easier to beg for forgiveness for not meeting lofty ambitions than to ask permission to fail, so sales will continue to attempt to hit an unrealistic target.
It should also be noted that bias is not always driven by human intervention; in times of prodigious growth, statistical forecasts based on historic orders will tend to forecast low. During rapid slowdowns, statistical methods tend to forecast high.
However, just like measuring forecast accuracy, measuring forecast bias is fraught with difficulty. At an aggregate or financial planning level, it is easy to understand and grasp. However, to get an accurate picture, we need to look at systematic bias at an SKU or forecast object level.
The overall result is invariably a KPI that is difficult for those outside of demand planning to understand. This is a serious problem. Gartner, the supply chain advisory firm, believes that the quality of the demand plan is the most important supply chain KPI. But a KPI that is not easily embraced or appreciated undermines the ability of the supply chain to communicate the performance – and importance – of the demand plan to their colleagues in other functions in the company. This is especially relevant when more and more companies aim for touchless flows, including demand forecasts, but come from a tradition of frequent forecast overwrites.
A more recent addition to the forecast KPI toolkit is Forecast Value Add (FVA), which measures how much a process or planner improved the accuracy of a forecast. 
The starting point is a naive forecast. A naive forecast could be as simple as an average, or what was sold last month. For more sophisticated organizations a naive forecast could be a regression with applied seasonality. The next step is to compare the actual demand with the naive forecast, and then compare the actual demand with that resulting from the planning process and/or IT system. The final step is to compare the difference between the accuracy of the naive forecast and the accuracy of the planning process. This needs to be done at an SKU or forecast object level.
A typical result is that good demand planners can add a lot of value to a small number of the items being forecasted, however for the majority, often 80% or more, intervention by planners reduces the accuracy of the forecast. Understanding FVA is a way to ensure that demand planners are identifying forecasts where they can add value.
Applying FVA can also help to make the planning process more effective and more efficient. Where planner intervention does not add value, organizations can rely more on the planning IT systems to create the best forecast, planners can focus more on those products where they are adding greater value.
FVA serves as a narrative tool to illustrate the value and efficiency of the planning process over time.
FVA may well be a way to turn the challenge of capturing the performance of the demand planning process without conflating it with the accuracy of the demand plan. 
One plausible reason demand planning KPIs are seen as complex and nebulous from the outside is that they are conceived and used by supply chain managers, who are almost always a highly analytical and numerically literate cohort. The search for the perfect demand planning KPI doesn’t yield a perfect result, it yields a KPI that tries to do too much. It is easy to lose sight of the narrative value of a KPI and its ability to tell a story that sheds light on performance. Yet narratives are not a natural inclination for supply chain managers.
FVA serves as a narrative tool to illustrate the value and efficiency of the planning process over time. All other things being equal, if the FVA is high, it validates and underscores the need to continue with the sales and operation planning. Should FVA decrease over time, the likeliest explanation is that the naive baseline method selection is improving, with automation doing more of the work of defining an accurate demand plan. Should FVA increase over time, the likeliest explanation is that the demand planners are wisely using their time to focus on the SKUs where they can provide the most impact.
Seen this way, FVA can be a valuable managerial tool, offering compelling storytelling to a dry, analytical issue. It is not a substitute for demand planning accuracy and bias. These KPIs are still critical and serve as analytical tools to understand where and why the demand plan is poor and can be improved. But these uses are more internal to the supply chain, wielded by the experts for themselves.
As an added benefit, if FVA can reflect positively on better automated baseline selection, that implies that it can help justify the case, and capture the value of eventual AI (artificial intelligence) solutions for demand planning. A test of an AI tool that lowers FVA provides the business case for the ability to deliver the same quality of demand plan with fewer planners. A true supply chain KPI for the future.
Forecast Bias and Forecast Value Add are functional metrics, which can help improve the overall accuracy of the forecast.
Ultimately, Forecast Accuracy is the one top-level metric that organizations care about. Companies that can forecast more accurately tend to have better service, lower costs, and lower inventory and are arguably more resilient. Forecast Bias and Forecast Value Add are functional metrics, which can help improve the overall accuracy of the forecast. Because of this, demand planners and data scientists, who are fine-tuning the planning tools, should be incentivized against how much they can improve the forecast and how successful they are at eliminating bias.
Professor of Operations Management at IMD
Ralf W Seifert is Professor of Operations Management at IMD and co-author of The Digital Supply Chain Challenge: Breaking Through. He directs IMD’s Leading the Future Supply Chain (LFSC) program, which addresses both traditional supply chain strategy and implementation issues as well as digitalization trends and the impact of new technologies.
Supply chain researcher, consultant, coach and lecturer
Richard Markoff is a supply chain researcher, consultant, coach, and lecturer. He has worked in supply chain for L’Oréal for 22 years, in Canada, the US and France, spanning the entire value chain from manufacturing to customer collaboration. He is also Co-founder and Operating Partner of the venture capital firm Innovobot.
Supply chain thought leader
Matthew Spooner is a supply chain thought leader and recognized supply chain visionary with deep industry expertise. He held supply chain leadership positions at ABB and Ericcson . At Gartner he was responsible for supply chain planning research. At Kinaxis, a leading supply chain solutions provider, he headed industry thought leadership.Â
27 March 2025 • by Robert Earle, Karl Schmedders in Strategy
Subsidies for renewables have led to electricity prices frequently falling to less than zero, creating opportunities for consumers. ...
26 March 2025 • by Didier Cossin, Yukie Saito in Strategy
Hannele Jakosuo-Jansson, Executive Vice President of People and Culture at Neste – the world’s largest producer of renewable diesel and sustainable aviation fuel (SAF) – and a board member of Finnair, has...
24 March 2025 • by Richard Baldwin in Strategy
It may feel like Donald Trump is capsizing the geopolitical boat, but could he just be accelerating a longer-term trend in the balance of global power? Geopolitics have long been heading for...
20 March 2025 • by Michael D. Watkins in Strategy
An intelligent organizational sensory system can detect, process, and act on signals of change, giving your company a powerful competitive edge. ...
Explore first person business intelligence from top minds curated for a global executive audience